As the use of self-service analytics continues to grow, so do the challenges associated with it. With more and more data coming in from a variety of sources, organizations must ensure they are equipped to handle it all. It is therefore essential that businesses have the right strategies and tools in place to make sure their data is managed correctly. In this article, I will discuss some of the most common challenges faced when dealing with self-service analytics and provide tips on how to overcome them most efficiently.
1. Introduction
Currently, the global business intelligence adoption rate stands at 26%, with 54% of enterprise leaders saying cloud BI is either very important or critical to their current and future initiatives.
With the rise of self-service data analytics tools, the BI penetration rate will undoubtedly continue growing. Still, not all businesses know how to use these solutions to their full advantage, struggling to resolve common usage issues. In this piece, I shed light on some of the challenges and how you can tackle them most efficiently.
2. Why is self-service analytics important for modern business?
Each business department from marketing, sales, and finance, all the way to supply chain management, generates data. While it creates enormous market potential, the larger the organization, the harder it becomes to derive insights and use them to the company’s advantage. Every time a team member needs to utilize data in their work, they ask IT for help to ‘pull’ data from the system. Over time, IT finds themselves overwhelmed with requests from within the company. Due to a lack of time and resources, they’re unable to keep up with the demand.
Given the above, it’s hardly surprising that the average large organization uses no more than 3% of its gathered data for business intelligence purposes.
Without a single, integrated source of data, business insights are siloed. Not only is data hard to find; it might be spread across multiple systems, making it impossible to put all elements of the informational puzzle into one, comprehensive view or dashboard.
Self-service data comes as a great solution to these challenges. It means that your staff members, irrespective of the department they work in, know where to access data and how to use it. This can mean anything, from collecting more intricate information for reports and prognoses to learning about overlaps between departments, boosting profitability, and many others.
A real-life example of obstacles in self-service analytics
At C&F, we’ve seen first-hand just how detrimental the lack of the right implementation and self-service usage guidelines can be. A Fortune 500 company introduced self-service features for reporting. At the moment of writing this post, they were in the process of migrating their data from a warehouse to a cloud solution.
Although they have, theoretically, already implemented some self-service practices, they still struggle with the following challenges:
- a lack of visibility of the number of reports, including how they’re used and by how many staff members
- unawareness of how frequently the data is being used
- a lack of consistency in data presentation.
They are also set to experience a couple of additional issues in the initial period after migrating to the cloud. These are difficult to optimize the usage of their cloud resources and to control expenses.
I discuss some of the above obstacles in detail next.
3. Self-service data analytics key challenges
Despite the irrefutable advantages of self-service business intelligence, its implementation can pose challenges. While some of them might go unnoticed, others are observed and reported on by businesses. They most commonly are cost-, technology-, and operations-related. I discuss them in detail below.
Eliminating data silos
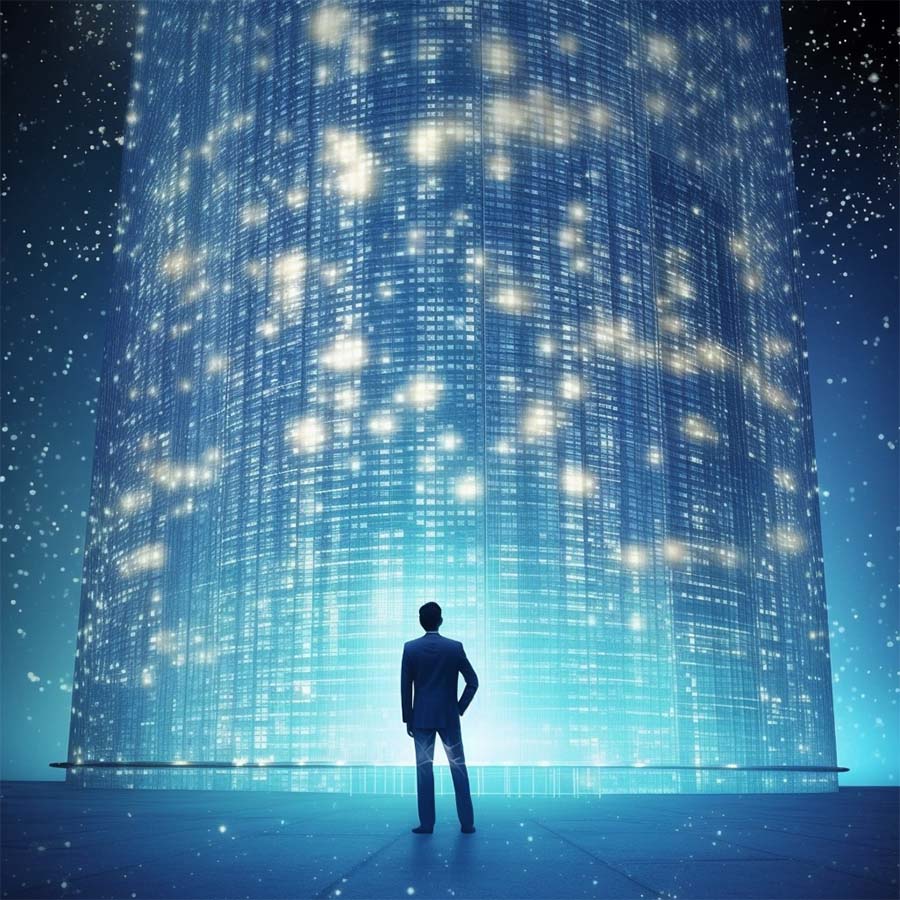
As mentioned above, most businesses experience data silos. These result from each department storing their data in various databases. Even if the teams share their data with each other, they usually create numerous copies of the same document or record. Not only does this introduce chaos. It also makes it harder to control:
- who uses the data,
- how it’s processed, and
- whether the company is compliant with data governance regulations (particularly, if they process personal and highly sensitive data).
Ultimately, employees lack a bird’s eye view of what kind of information they have at their fingertips. Even if they do come across relevant data, they don’t know which version of the data set is most accurate, complete, and reliable for data decision-making.
Navigating business risk management
One of the most significant risk factors is whether you can trust the data you see.
Throughout the years we’ve seen many situations where incorrect information wreaked havoc on entire organizations. If too many employees are given access to the data, they might start modifying it and using the information to run their own calculations or reporting.
Even if the data they used was valid, they might make an error in their calculations. The risk is particularly high for those who aren’t tech-savvy or don’t understand the wider context, i.e., where the data originated from. As a result, everyone might get different results.
The costs of wrong insights are multi-faceted – not only does your business lose money on generating incorrect analyses. If these assets are used as proof of concept for business changes or introducing new services, you’re facing a risk of a disaster – one that could threaten the entire enterprise.
The above scenario proves that self-service analytics must always come paired with careful data access and edit control. The more mistakes and wrong data interpretation instances, the higher the risk of low quality data. Instead of treating your business intelligence tools as a single source of truth, employees will question the validity of others’ findings. This, in turn, might result in a cross-organizational drop in productivity, which I explore further in this post.
Keeping costs under control
This issue applies specifically to platforms, which offer usage-based pricing such as Tableau or Power BI or even a layer below where the data is processed. Employees are free to use them how and when they please. There are no usage guidelines in place. What often happens is that users run the same queries as their fellow team members without realizing they can already access the results and each query needs to be paid for.
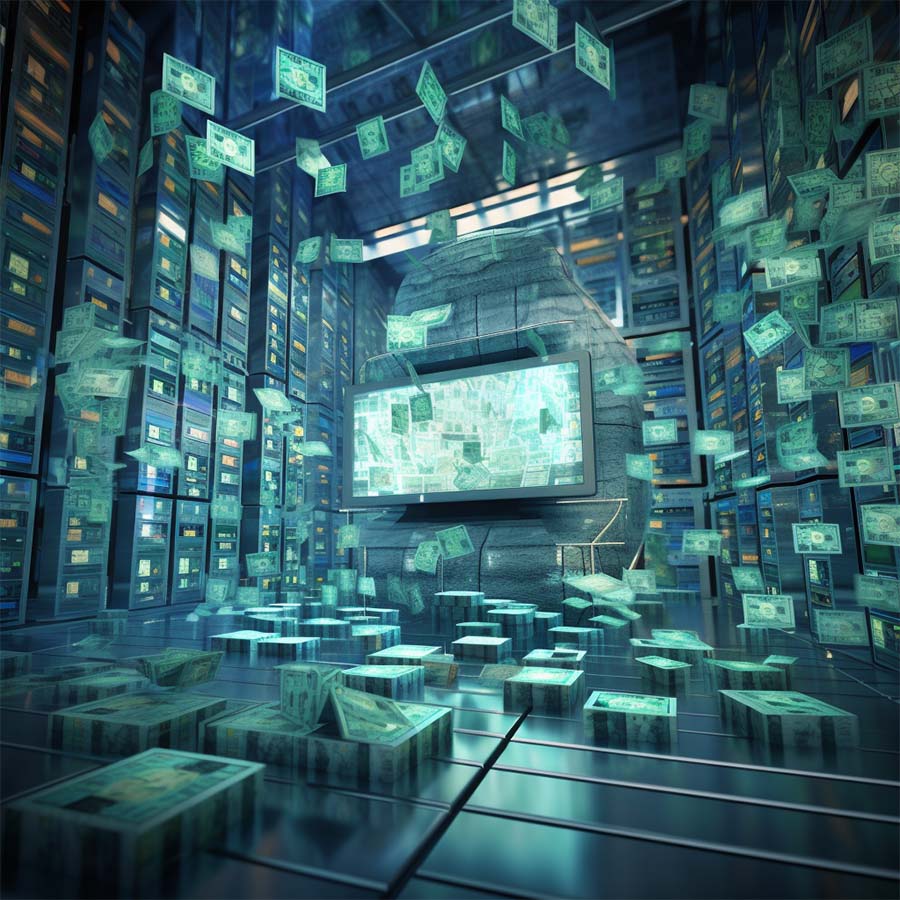
Additionally, people can generate multiple versions of the same report with minor changes instead of simply updating the current copy. What happens is that companies end up with hundreds of reports a week with very few employees using them due their limited application. This results in:
- extra costs for the company
- lots of unused datasets, which create chaos
- limited cooperation between user groups, as people constantly redo the same reports, instead of reusing the existing ones.
One of the goals of self-service analytics is to identify at the organizational level the reports, and use cases that should be centralized and distributed in a more deliberate and controlled manner. This helps with significant cost reduction not only because it enables people with no tech expertise to access data but also because it improves data quality and reliability.
Basing decisions on incorrect data or data you cannot trust can have severe business consequences, the fixing of which can generate massive costs.
Maintaining high employee productivity
High productivity calls for a lack of chaos. Employees who use data on a daily basis prefer clean, legible data environments. If they notice that data and reports are chaotic, they become discouraged, disengaged, and might even lose motivation to perform the work.
There’s also the issue of navigating and discovering data. Some users, particularly those who aren’t tech-savvy, might be tentative in their approach to self-service analytics. They might worry they won’t be able to find the data by themselves. And even if they do, they might wonder if the data is correct and if they’re making the right decision based on it.
Alternatively, they might decide to ask a team member who’s more tech-savvy to help them. However, this isn’t how self-service analytics should work. After all, it’s about data democratization. That’s why, before introducing a new self-service platform, each employee should go through training to be able to confidently use it.
Avoiding chaos in data and reports
Chaos in data is more of a symptom of an issue than a problem itself. It’s often the result of a wider, underlying issue. Most commonly, the culprit is either data silos or lack of data usage control, both of which were discussed above. On that note, there’s also another aspect – one that might not be as easily quantifiable but as important – the organization’s image.
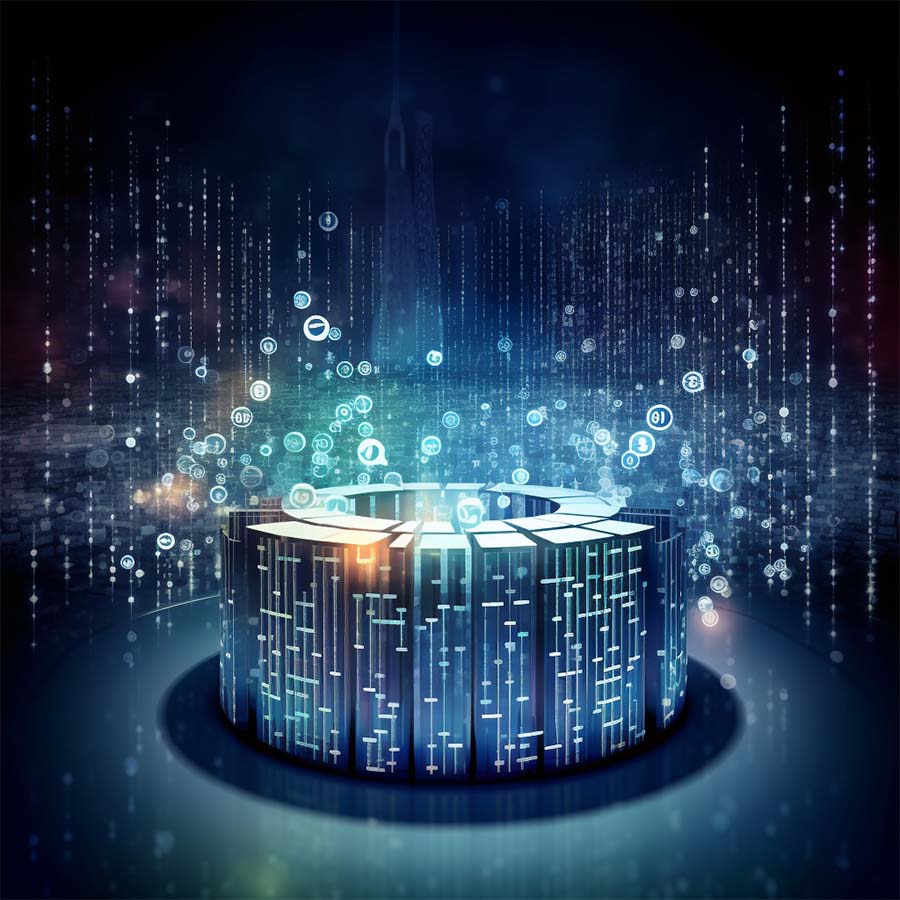
If a team member has to use unstructured, chaotic data daily, they quickly realize that it takes up tens of hours each month to derive information that should be highly accessible and organized. This, as a result, impacts their perception of the entire business.
For instance, imagine that a manager wishes to demonstrate the results of implementing a new process at a meeting with the C-suite. It wasn’t part of their presentation, nor have they downloaded it in a PDF file, but they know that they can easily access the data in the tool. After logging in, the manager discovers that the report they’ve created has been edited or, worse yet, moved elsewhere. Such an event reflects negatively on the manager’s professional image, leading to stress or discouraging them in their work. It’s hard to imagine an organization thriving if data analytics are kept in disarray.
4. Mastering self-service data analytics
A well-designed and managed self-service analytics program helps businesses make better decisions and quickly respond to market fluctuations and demand. The key to making the implementation a success is partnering up with the right expert. At C&F, we can:
- run an audit of your existing data landscape and help you choose the best tools and approach, which will ideally reflect your business use cases and unique needs
- help you implement your self-service tool stack
- offer employee training and onboarding.
Reach out and let’s discuss how we can support you on your path towards data democratization.