Introduction
Back in 2020, the global AI market in the life sciences industry was valued at $1.3 billion by Grand View Research. It is expected to have a compound annual growth rate of 41.2% between 2021 to 2028. This positive outlook is attributed to more than the pandemic; it’s also a result of increased adoption of AI in areas like disease diagnosis, personalized healthcare, and drug discovery.
Given the efforts that LLM creators, including OpenAI, are putting into boosting the accuracy and capabilities of their engines, AI has the potential to revolutionize the healthcare sector at an unprecedented pace. Its impact, however, also depends on whether AI meets the strict privacy and security legislation requirements that go hand in hand with analyzing clinical data.
In this article, We are going to take a closer look at how AI is impacting the life science industry.
How AI is changing the life science industry
Few industries are experiencing as strong an impact of AI as life sciences. On top of improving communications and simplifying administrative work, which is a common benefit across all industries, AI is also affecting the supply chain and research quality. This, in turn, is bringing the world of healthcare into a whole new era.
Artificial intelligence and machine learning help life science businesses draw more insights from their data, ideate business model improvements, and accelerate product development.
“First and foremost, AI grants us the possibility to implement solutions, which can elevate data analytics to a previously unattainable level”, says Maciej Kłodaś, C&F’s Head of UX. “Instead of analyzing Business Intelligence reports manually, we can query the data source for specific information, either through voice or text search. Or, we can go a step further, and ask it to generate a dashboard that showcases the areas we’re interested in, on the spot. This is the next step in the mass adoption of BI solutions; one that undoubtedly lowers the entry barrier for organizations which represent a lower degree of analytics expertise”.
With that in mind, below are some of the most significant developments in the medicine, pharma, and broader life science field.
Drug discovery and research
Drug discovery is a long and time-consuming process. Luckily, it can be completely revolutionized by AI. By applying machine learning, molecular complexities can be decoded quickly, allowing researchers to reveal connections between treatments and illnesses. This can lead to the development of new, innovative therapies, drug repurposing, and potential cures. What’s more, AI encourages collaboration on global research – it enables cooperation between researchers and institutions, which positively impacts research outcomes.
Drug development
Drug development is another process that is about to be transformed thanks to the use of AI – it will become a lot faster and more efficient. By analyzing data from genomics, proteomics, and clinical trials, Artificial intelligence can suggest potential drugs, which streamlines validation. How exactly? It can predict how effective and safe to use the drug will be. This results in significant time savings – when it comes to both preclinical and clinical testing.
All of this has a positive impact on promoting precision medicine i.e., offering therapies and drugs tailored to specific patient groups. However, in order to reap the full benefits of AI in drug development, we first must address the concerns on data privacy and ethics. These call for an introduction of the right regulations.
Personalized medicine
More often than not, when it comes to drug dosing, the same approach is used with all patients – it’s usually based on a patient’s weight and age. This can be modified thanks to artificial intelligence, which continuously monitors how a patient is responding to a drug and uses the findings to suggest a personalized drug dose that proves more effective. Unlike the conventional approach, AI considers a patient’s individual characteristics, responses, and other factors like genetic mutations.
Helping with regulatory compliance
The ability to automate data monitoring and analysis is great news to pharmaceutical firms and medical device manufacturers as it might help them with meeting regulatory standards. Incomplete documentation can give grounds for legal actions and penalties, which extends the drug’s launch time. AI systems can spot compliance issues and let regulatory teams know about them so they can address them immediately.
Running clinical trials
The amount of data generated during clinical trials is vast. Tufts University reports that Phase III protocols collect an average of 3.6 million data points in the post-pandemic reality. This is about three times as much as tests conducted a decade ago. Using AI to automate data collection, processing, cleaning, and analysis can accelerate work and minimize the costs of the study.
Improving patient outcomes through better communication
With AI, companies in the sector can now analyze patient data collected from on-site medical devices, wearables, as well as self-reported symptoms. By accessing all the intricate information, AI-powered technologies can detect any anomalies and, if need be, recommend better courses of action. It can also be used to improve the perceived level of care and safety for the patient. This can be very important, particularly when patients are part of previously mentioned experimental procedures.
A great example are AI chatbots, which can be used alongside telehealth solutions. An AI chatbot can access available patient data and complement it with information collected through an individual. By analyzing all of the available data, the chatbot can either provide a recommendation or scale the situation at hand to a human scientist or doctor.
At C&F, we’re currently working on developing user-friendly solutions and consumer applications for the animal health sector. These will be based on AI models, which will take the form of chatbots that will help pets communicate with their owners. Thanks to our application, pet owners will be able to identify troubling symptoms, get potential solutions, or receive information that a vet visit is necessary.
Building better relationships with clients
Life science businesses can use AI to analyze their target customers’ goals, needs, and challenges, and use these insights to develop products that perfectly meet these objectives. This is possible through AI’s natural language processing capabilities.
Additionally, companies in the pharma and healthcare sectors can use it to refine their marketing and sales strategies. Among others, AI can help them:
- Analyze the performance of advertising channels
- Detect anomalies in customer behavior or the completion of KPIs
- Segment leads and clients to tailor their communication.
Examples of advanced AI implementations in life science
- Drug development made faster and safer
On average, it takes between 10 and 15 years to develop a drug. This process is very complex and involves extensive testing, which explains why it takes so long.
But what if we could use AI to represent every single body cell? It would function like a virtual cell, perfectly mimicking human cells. Scientists could then use it to test cells’ reactions to infections, diseases, and drugs.
This would massively speed up patient diagnosis and treatment, making the process of new drug discovery much quicker and more efficient. It’s not a fictional scenario; quite the opposite! A virtual cell modeling system is coming into life thanks to Priscilla Chan and Mark Zuckerberg.
- Better visual diagnostics
One of the most powerful capabilities of AI in health-related industries is computer vision, where data is extracted from images and video. While initially used for purposes like facial recognition and sports analyses, the technology has gotten so precise that it’s now becoming an integral part of radiology.
One of the more promising developments of 2023 is a technology called PANDA, short for pancreatic cancer detection. Scientists have tested the AI’s capability to properly detect pancreatic cancer by analyzing CT scans and compared it to the level of precision offered by human specialists. Results shared by the scholars behind the solution indicate that it is 99.9% correct in recognizing cancer-free images, and 92.9% in those with cancer.
These types of solutions can be used by life science companies to properly diagnose and qualify patients for experimental treatments. In the long run, it could also support large-scale population analyses to detect disease in its pre-symptomatic phase.
- Automated pharmacovigilance
Pharmacovigilance (PV) is an area that aims to detect drug-related, adverse effects. Traditionally, it involves significant human power to constantly monitor patients, spot, and investigate any symptoms that might indicate side effects of a drug in development. Perhaps, most importantly, PV should provide instructions on how patient safety could be enhanced by improving the drug formula or treatment routine. AI now relieves PV specialists of the need to peruse data manually, minimizing the risk of mistakes in side effects detection.
There are several AI-powered systems on the market that make it easier to run quality assurance checks, as well as detect the potential toxicity of new drugs at a pace impossible for PV protocols that are handled entirely manually. Their efficacy has already been proven by studies conducted by pharmacology experts.
- Bionic engineering
AI now functions as the missing element of the puzzle between artificial, hardware limbs and patients’ nervous systems. The life science industry is already testing prostheses that can communicate with patients’ brains and respond to signals for movement.
One of the latest examples of where the technology is heading thanks to AI comes from Bionics Institute. A patient, who suffered a below-elbow amputation several years ago, has been using an AI-powered artificial limb controlled with the help of AI through a neuromusculoskeletal implant. As of late 2023, they were able to use their bionic hand to an extent that ‘somewhat’ resembles that of a human hand.
The goal is to develop a technology that will not only work with the person’s body, but also trigger movement at a place close to that which happens with natural limbs.
5. Clinical trial matching
AI can also help speed up and automate finding suitable patients for clinical trials. In the pre-AI era, proper recruitment could take months if not years, often resulting in a fiasco. In fact, one study found that 86% do not meet enrollment deadlines.
The use of artificial intelligence in the eligibility analysis can speed up the pre-qualification process immensely. Some of the particularly helpful technologies include computer vision, which let the AI read text stored on images like medical records or CT scans.
Once patients have been onboarded, AI can monitor patient’s health through all of the clinical trial stages. The ability to look at all patients at scale enables effective resource allocation, prioritizing the most critical cases. This not only reduces costs, but, most of all, enhances patient care. As potential problems are identified early, thanks to the use of AI, the chances of curing patients are higher.
Creating your own GPT in life sciences – is it worth the effort?
If you don’t know it yet, you can create your own GPTs now; anyone can, as it requires no coding! You can use it to assist you in daily life, with specific tasks, or build one to help you at work. It’s very simple to do – all it takes to bring it to life are a few instructions of what it should do, and you can select the actions you’d like it to take like, browsing the web, analyzing data, or making images. Then hit publish and it’s ready to use.
Some of the benefits you can expect by creating your own GPT as a life science company include:
- Customization for Industry-Specific Language: life science businesses are full of jargon, terminology, or even domain-specific language. You can build your own GPT and train it to use the vocabulary that fits your business. It will be able to create content that perfectly resonates with your audience, as it will be industry-specific.
- Tailored information extraction: there is a lot of data going around in the life sciences sectors, a huge part of which is unstructured, including clinical trial reports, scientific literature, and patient records. You can train a GPT to extract relevant information, which will significantly speed up the process of data mining and knowledge discovery.
- Enhanced compliance and security: Handling sensitive information, like patient records, calls for special security measures. By building your own GPT you can guarantee better control over data privacy and security by ensuring compliance with specific requirements.
- Ability to adjust it to company-specific queries: As each life science company has its own portfolio of drugs and clinical studies results. Using a domain-specific GPT could be an extremely useful source of information. Instead of referring to numerous internal databases, scientists could run a query and receive a comprehensive answer that draws data from all the information systems.
- Enhanced clinical decision possibilities: Understanding the results of a clinical study, including any reported adverse effects or outcomes, is critical in deciding whether a drug meets the criteria needed to bring it to the market. Creating a custom NLP model lets decision-makers refer to internal data like patient records as well as any external sources the company deems important, for instance, medical research from scientific centers.
- Minimized need for using external APIs: Building your own GPT and prioritizing your first-party data can also bring down the necessity to integrate with third-party channels. The organization can prioritize its domain-specific findings and focus on external APIs from groundbreaking, open-sourced research only. Relying on owned data means a lower risk of losing access to research data.
How intelligent is ChatGPT / GPT4
The new version of ChatGPT, i.e., ChatGPT4 generates a mix of emotions – excitement and fear. You can ask it to do plenty of things, like explain complex scientific phenomena, write birthday wishes, write you a song, or a letter to a CEO. If you think it’s pretty smart, then you’re right. In fact, it got a B minus in assignments at Wharton’s MBA exam, 710 out of 1000 on SAT, and it also performed very well on LSAT. That’s pretty impressive.
How is the new version different from the previous one?
To begin with, it’s able to deal with more complex instructions, it can answer any question, and much more accurately than GPT3.5. Experts say that it’s 5 times better than its older brother – it’s powerful enough to handle a lawsuit, which is equally terrifying and exciting!
And you know what they say – with great power comes great responsibility. That’s why it’s key to put in place AI legislation that would guide governments, corporations, academic researchers and citizens towards the right use of AI, emphasizing respect for user’s privacy and security. And it has to happen quickly.
The AI is developing incredibly fast, faster than anyone has thought it would. While it brings many benefits to the society, it’s not free from negative implications, such as job losses, privacy breaches, copyright infringements, etc. If humans don’t start to control it, the consequences can be catastrophic.
Even the father of OpenAI Sam Altman, back in 2015 said “super human intelligence is probably the greatest threat to the continuous existence of humanity”. Although he repeated the same statement in 2023, it hasn’t stopped him from further developing chat GPT.
How capable are GPT models in handling medical and scientific data?
Without a doubt, GPT models have been making vast improvements when it comes to their ability to analyze healthcare data.
Microsoft reported that it has been testing the capabilities of GPT4 against the same standards as those used to evaluate professionals in the medical field. The prompting strategy, called Medprompt, was developed on the basis of the MedQA dataset. In late 2023, the AI was able to achieve a few milestones, such as getting a 90% correct score when tested on the knowledge stored in the MedQA dataset for the first time. It also demonstrated a 27% lower error rate than MedPaLM 2. Below is a graph that demonstrates how the scientific data analysis accuracy improved in fewer than a year, i.e., between the launch of Chat GPT on November 30th, 2022 and September 2023:
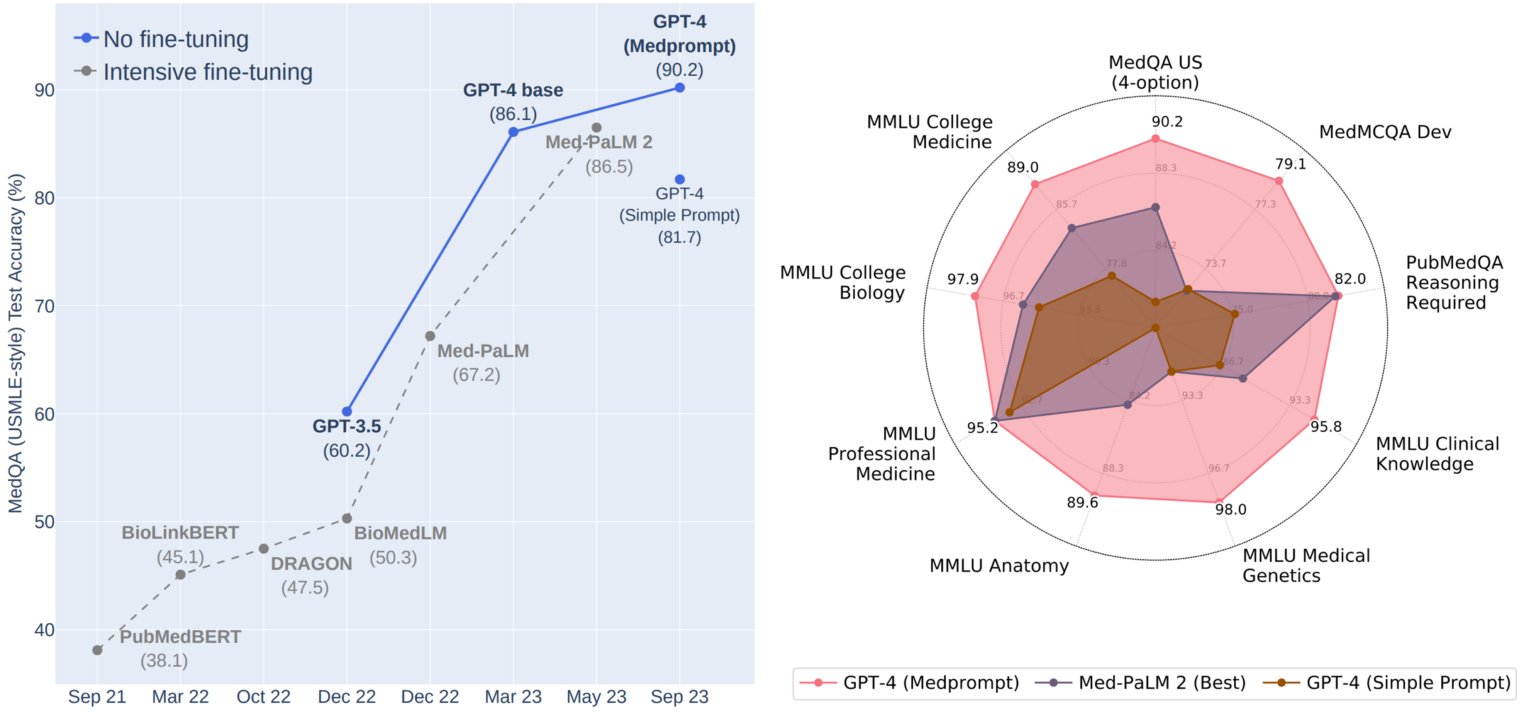
/Image source/
But does that mean that life science and medical organizations can now confidently expedite all the analysis to GPT4 based models? Not quite, as humans should remain in the loop; after all, it’s an LLM, and not a real-life clinical expert.
Scientists and data professionals should regularly audit the output and accuracy of GPT4-based models. There have been reports of inaccurate information generated by the latest version of GPT, particularly in niche disciplines. In an industry as critical to the well-being of populations as life sciences, any AI-generated data should be verified by human experts. That said, not all errors will result from GPT’s lack of capability. As in all other fields, the quality, structure, and completeness of the data you input will also impact the relevance of the model’s output.
AI revolution – what does the future hold?
With the introduction of GPT models, AI has now been fully democratized. It’s present in every industry, irrespective of the company size. In fact, it’s just a few clicks away for every household or on every mobile device.
That said, life sciences is one of the areas where the impact of AI is truly ground-breaking. Not only does it allow to automate repetitive work, like it can universally for any other industry. Its biggest value is that it can help scientists and researchers access and analyze data to significantly speed up drug development, introduce new treatment protocols, or even
develop bionic technologies. Think of things like bionic limbs powered through brain waves or caps that allow those who’ve lost their speech ability to communicate with the outside world – these are no longer reserved to science fiction models and are becoming a reality.
In order to use AI to its fullest capability, however, organizations need to ensure that the data they use is not only diverse, but also standardized and analyzed properly. Data accuracy should lie at the core of any AI project. In a field as critical to human lives as life science, its impact will reach well beyond company profitability.